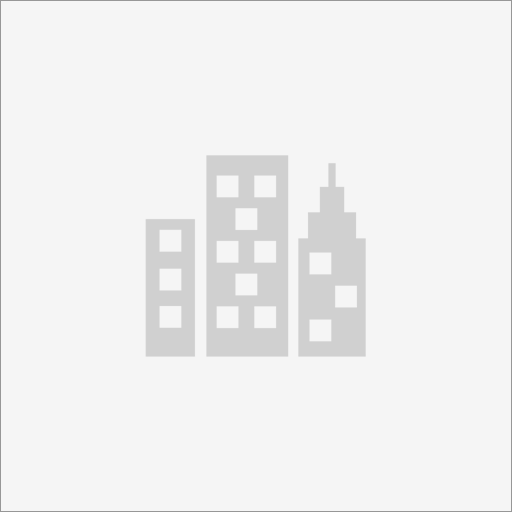
Imperial College London
Job title:
Research Associate in Deep Learning for Computational Lightfield Microscopy
Company
Imperial College London
Job description
About the role:Applications are invited for the above post to work with Prof. Pier Luigi Dragotti and his team at Imperial College London for a Wellcome Trust funded project.The successful candidate will be integral to delivering on the project called “Optical Oscilloscope: Real-time, High-throughput, Volumetric Voltage Imaging.” Our goal is to enable real-time, kilohertz, volumetric voltage imaging in 1,000 cells simultaneously within scattering mammalian brain tissue. The project is driven by a transdisciplinary consortium led by Dr. Foust (Imperial, Bioengineering), and includes Prof. Pier Luigi Dragotti who is an expert in machine learning, signal processing and computational imaging (Imperial, EEE), Prof. Christos Bourganis (Imperial, EEE); and Dr. Samuel Barnes (Imperial, Brain Sciences).The successful candidate will develop a new generation of computationally efficient, stable, and interpretable deep neural networks for volume reconstruction from lightfield video sequences produced by our lightfield microscope.What you would be doing:You will implement, test and optimize new model-based deep neural networks (DNN) for real-time, neural activity extraction from lightfield microscopy data. You will develop strategies to systematically embed prior knowledge and constraints about neural signals and image acquisition optics into the DNN architectures. Your neural networks will be robust to distribution shifts and will be trained in a semi-supervised fashion using small amount of training data. You will also work with postdoctoral associates in EEE and bioengineering to develop algorithms that will be implemented in a field-programmable gate array for real-time readout.What we are looking for:The successful candidate:
- Will have a PhD (or be very near completion, or equivalent) in engineering, mathematics, physics, or a related topic.
- Experience with developing model-based deep learning architectures and with developing algorithms for inverse imaging problems is required.
- The successful candidate will have a good publication record, show evidence of working well in teams, and demonstrate an ability to work to tight deadlines.
- Preference will be given to candidates with a strong background in signal/image processing and to those with experience with programming in Matlab, Python and/or C/C++.
What we can offer you:
- The opportunity to form a key part of the multidisciplinary team who will innovate methods for high-throughput, volumetric voltage imaging Human Resources Division
- The opportunity to form a key part of the multidisciplinary team who will innovate methods for volumetric voltage imaging
- Training in mathematical methods and algorithms for inverse problems and close interaction with the highly motivated and diverse team of Prof. Dragotti all working on imaging problems, deep learning and high-dimensional data analysis
- First access to spatially-resolved cortical microcircuit voltage data acquired on a previously inaccessible scale. Flexibility to develop your own algorithms and research vision based on this previously unaccessible data
Further InformationProf. Dragotti is an expert in mathematical methods for image/signal processing and he applies these methods to develop interpretable model based neural networks for computational imaging applications. He provides a diverse and supportive training environment promoting interdisciplinary research.This full-time, in-person postdoctoral position is based at Imperial College’s South Kensington Campus in London, UK, and is funded for 18 months initially, starting in November 2024.Closing date: 20st October 2024To apply, visit www.imperial.ac.uk/jobs and search by the job reference ENG03276£48,056 to £56,345 per annum
Expected salary
£48056 – 56345 per year
Location
South Kensington, South West London
Job date
Sun, 22 Sep 2024 02:58:52 GMT
To help us track our recruitment effort, please indicate in your email/cover letter where (jobs-near-me.eu) you saw this job posting.